The PredNet is a deep convolutional recurrent neural network inspired by the principles of predictive coding from the neuroscience literature [1, 2]. It is trained for next-frame video prediction with the belief that prediction is an effective objective for unsupervised (or "self-supervised") learning [e.g. 3-11]. The PredNet architecture is illustrated below. An animation of the flow of information in the network can be found here.
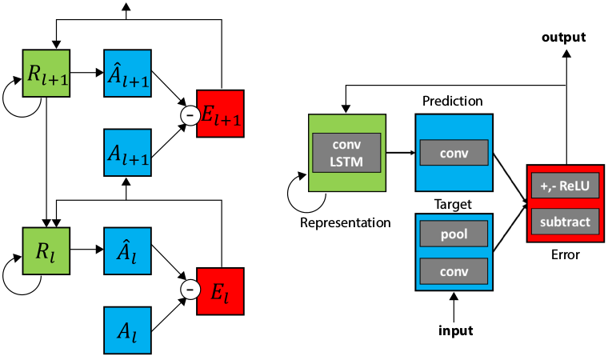
Next frame predictions on the Caltech Pedestrian [12] dataset are shown below. The model was trained on the KITTI dataset [13]. See the repo for downloading the model.
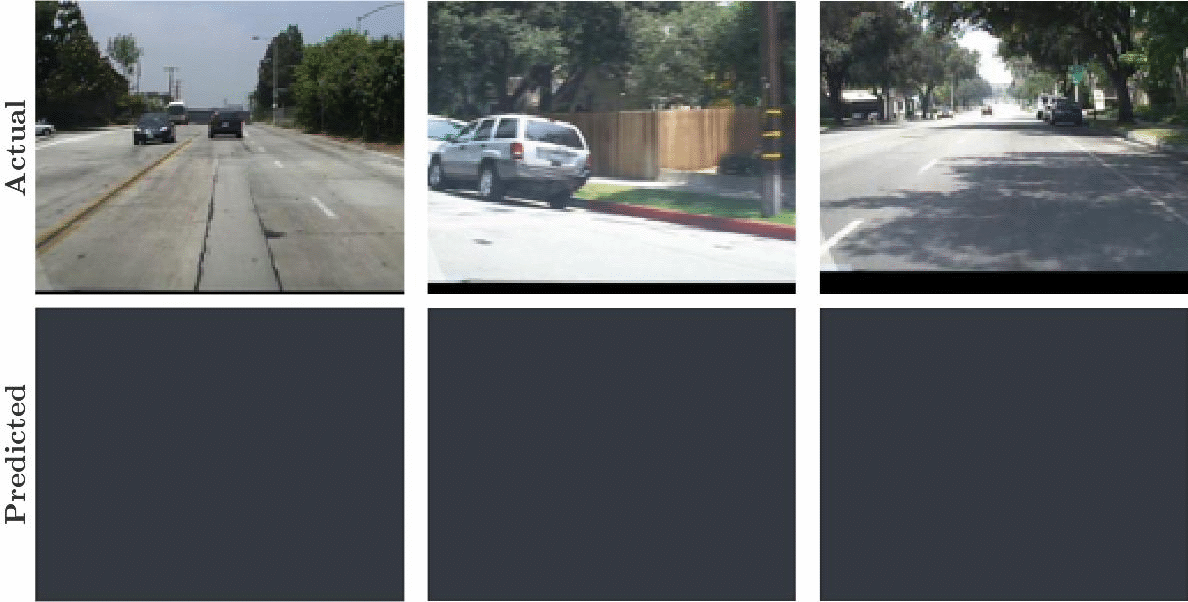
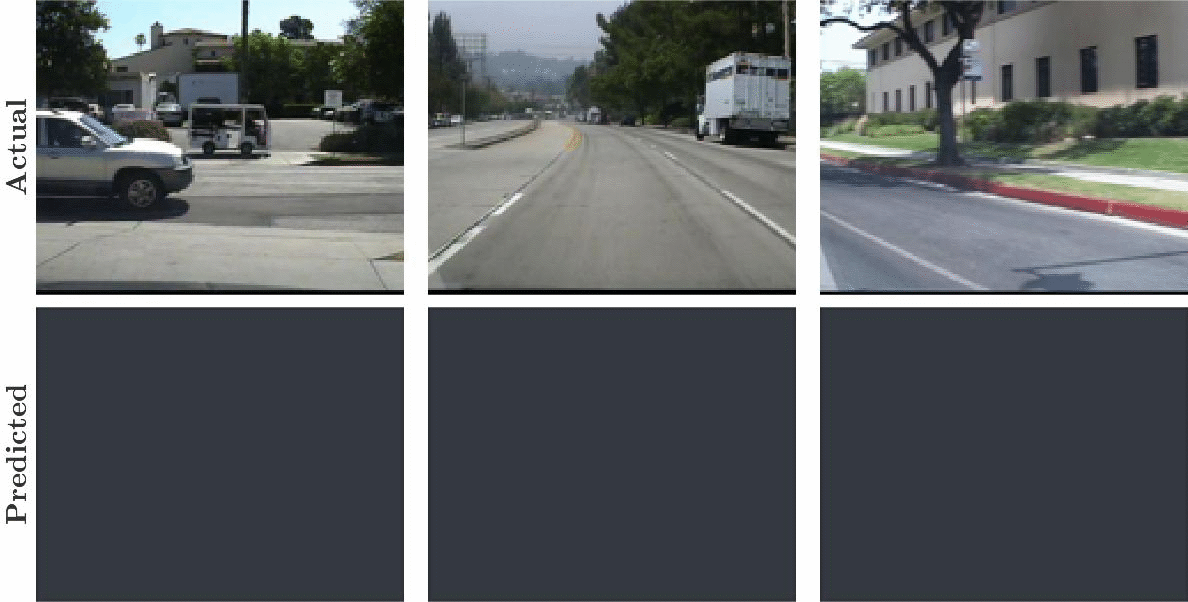
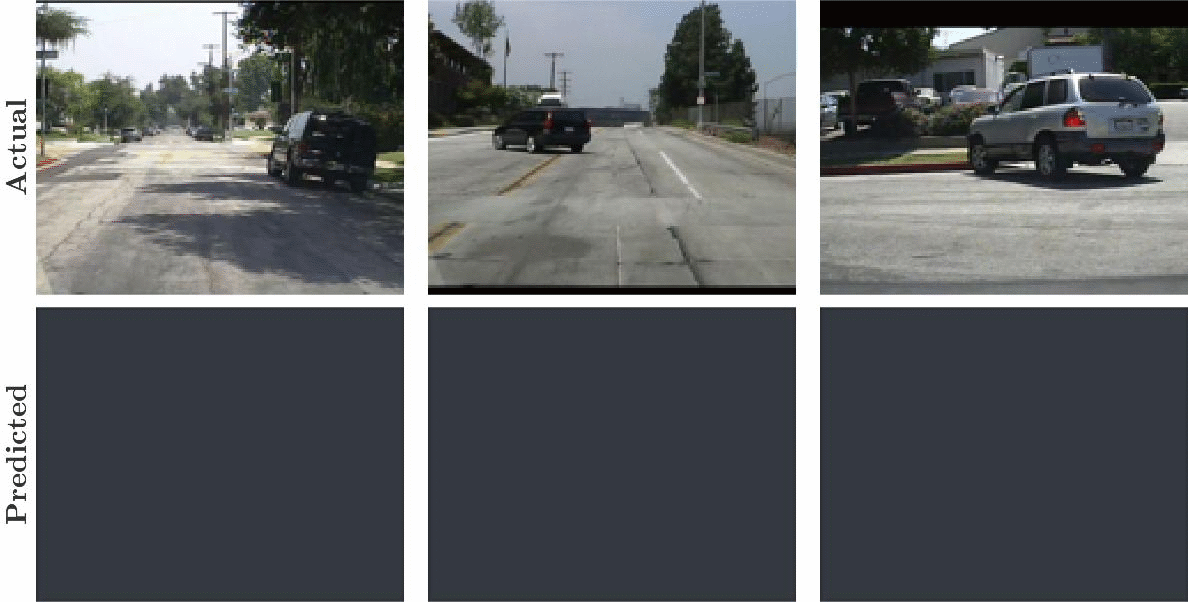
Multi-timestep ahead predictions can be made by recursively feeding predictions back into the model. Below are several examples for a PredNet model fine-tuned for this task.
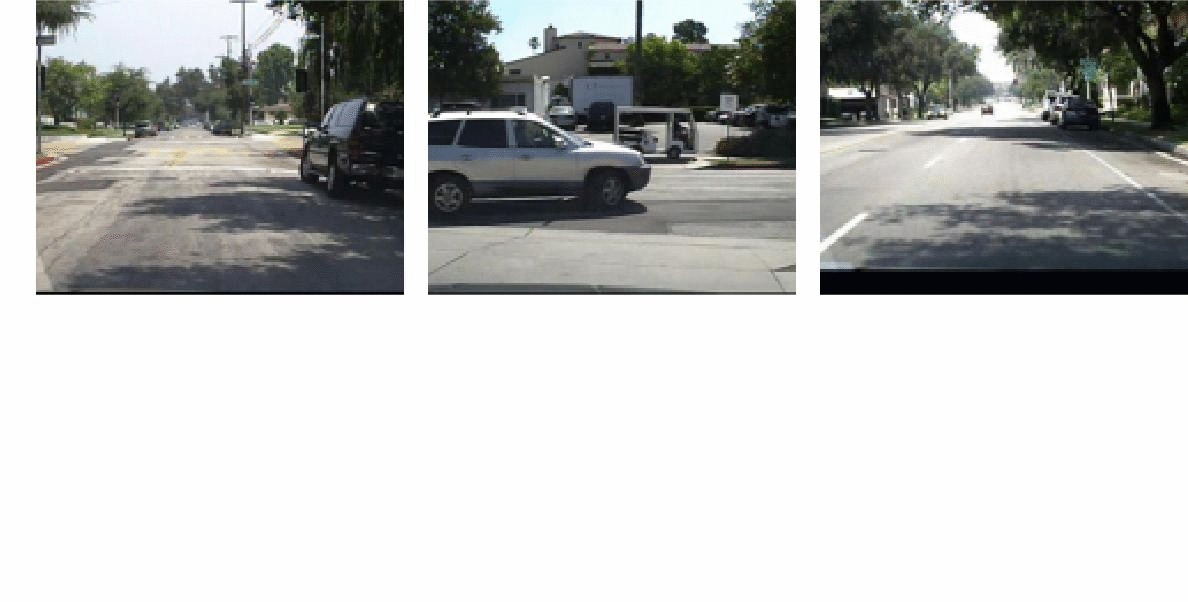
- R. P. N. Rao and D. H. Ballard. Predictive coding in the visual cortex: a functional interpretation of some extra-classical receptive-field effects. Nature Neuroscience, 1999.
- K. Friston. A theory of cortical responses. Philos Trans R Soc Lond B Biol Sci, 2005.
- W. Softky. Unsupervised pixel-prediction. NIPS, 1996.
- D. George and J. Hawkins. A hierarchical bayesian model of invariant pattern recognition in the visual cortex. IJCNN, 2005.
- R. B. Palm. Prediction as a candidate for learning deep hierarchical models of data. Master’s thesis, Technical University of Denmark, 2012.
- R. C. O’Reilly, D. Wyatte, and J. Rohrlich. Learning through time in the thalamocortical loops. arXiv, 2014.
- N. Srivastava, E. Mansimov, and R. Salakhutdinov. Unsupervised learning of video representations using lstms. arXiv, 2015.
- R. Goroshin, M. Mathieu, and Y. LeCun. Learning to linearize under uncertainty. arXiv, 2015.
- M. Mathieu, C. Couprie, and Y. LeCun. Deep multi-scale video prediction beyond mean square error. arXiv, 2015.
- W. Lotter, G. Kreiman, and D. Cox. Unsupervised learning of visual structure using predictive generative networks. arXiv, 2015.
- V. Patraucean, A. Handa, and R. Cipolla. Spatio-temporal video autoencoder with differentiable memory. arXiv, 2015.
- P. Dollár, C. Wojek, B. Schiele, and P. Perona. Pedestrian detection: A benchmark. CVPR, 2009.
- A. Geiger, P. Lenz, C. Stiller, and R. Urtasun. Vision meets robotics: The kitti dataset. IJRR, 2013.